What Are the Privacy Concerns With AI?
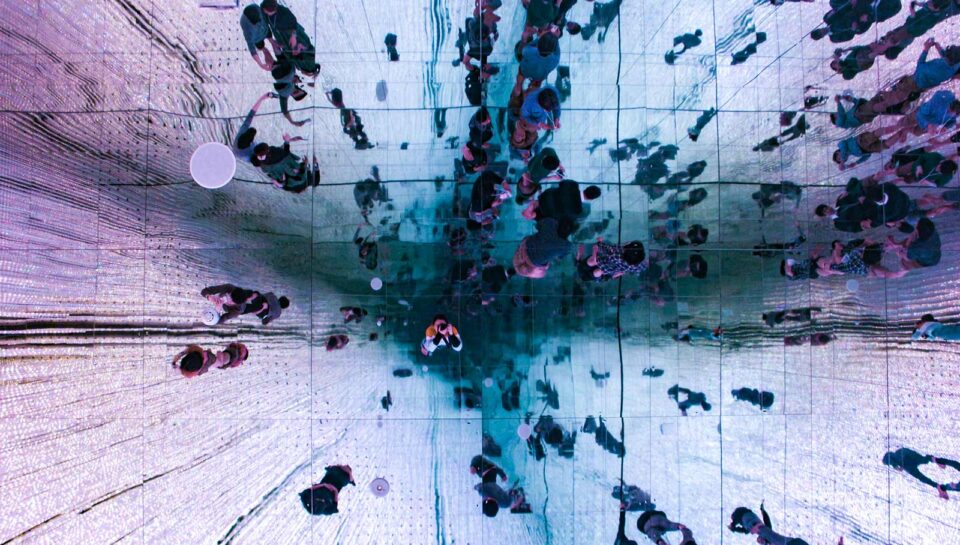
In an era where artificial intelligence is rapidly transforming every aspect of our lives, from how we shop to how we receive healthcare, questions about privacy have become increasingly urgent. As AI systems collect, process, and analyze unprecedented amounts of personal data, individuals, organizations, and governments are grappling with complex privacy implications that were unimaginable just a decade ago.
The Data Appetite of Modern AI
Modern AI systems, particularly machine learning models, thrive on data—and lots of it. The more information these systems can process, the more accurate and useful they become. This fundamental characteristic creates an inherent tension with privacy principles.
Training Data Collection
AI systems are trained on massive datasets that often contain personal information. Language models like those powering chatbots are typically trained on vast collections of text scraped from the internet, which may include forum posts, emails, social media content, and other sources where individuals have shared personal details without expecting them to be used for AI training.
Consider the case of GPT-4 and similar large language models, which have ingested billions of web pages, books, and articles. This training corpus inevitably contains personal information that was never explicitly contributed for AI development. While companies attempt to filter out sensitive information, the scale makes perfect filtering virtually impossible.
Operational Data Gathering
Beyond training data, AI systems continuously collect operational data during use. Voice assistants record and analyze speech patterns, recommendation systems track viewing or purchasing behaviors, and facial recognition systems capture and process biometric information. This ongoing data collection creates digital profiles of users that grow increasingly detailed over time.
For example, smart speakers like Amazon Echo or Google Home are always listening for their wake words, which means they capture snippets of conversation that may contain sensitive information. While companies claim these devices only begin recording after hearing the wake word, studies have shown they can be activated by similar-sounding phrases, potentially leading to unintended recording of private conversations.
Consent and Transparency Challenges
One of the fundamental principles of data protection is informed consent—the idea that individuals should understand and agree to how their data is used. AI systems, however, often operate in ways that make meaningful consent difficult to achieve.
Complex Data Processing
AI systems process data in complex, often opaque ways that can be difficult to explain in terms understandable to the average person. Privacy policies may disclose that AI is being used, but rarely provide sufficient detail about what specific data points are being analyzed, how they’re combined with other information, or what inferences are being drawn.
For instance, when you use a social media platform that employs AI for content recommendation, the system may analyze not just the posts you explicitly interact with, but also how long you pause on certain content, what time of day you’re most active, which friends’ content you engage with most, and countless other signals. The resulting profile is far more detailed than most users realize when clicking “I agree” to terms of service.
Secondary Uses of Data
Data collected for one purpose is frequently repurposed for secondary uses, particularly as companies seek to maximize the value of their data assets. Information provided to an AI-powered fitness app might later be used to train healthcare diagnostic systems or develop targeted advertising profiles.
A notable example comes from IBM’s acquisition of medical images for AI training. In 2019, it was revealed that IBM had obtained millions of medical images from academic institutions without clear consent from patients for AI development purposes. The images were originally collected for medical care but repurposed for commercial AI development.
Inference and Re-identification Risks
AI systems excel at finding patterns and making predictions, which creates unique privacy challenges even when data appears to be anonymized or aggregated.
The Fallacy of Anonymization
Traditional approaches to protecting privacy often rely on anonymization—removing directly identifying information like names or ID numbers. However, modern AI can often re-identify individuals by analyzing patterns across multiple data points.
Research has repeatedly demonstrated that supposedly “anonymized” datasets can be re-identified with alarming accuracy. In one famous study, researchers were able to uniquely identify 87% of Americans using just three pieces of information: gender, date of birth, and ZIP code. With more data points and sophisticated AI techniques, re-identification becomes even more feasible.
Sensitive Attribute Inference
AI systems can infer sensitive characteristics that individuals never explicitly disclosed. By analyzing patterns in seemingly innocuous data, AI can predict health conditions, sexual orientation, political beliefs, and other highly personal attributes.
For example, researchers have demonstrated that AI analysis of social media activity can predict depression with reasonable accuracy, potentially revealing mental health information that individuals never intended to share. Similarly, purchasing patterns can reveal pregnancy or other medical conditions before an individual has chosen to disclose this information to others.
Surveillance and Monitoring Concerns
AI dramatically enhances the capabilities of surveillance systems, raising profound questions about privacy in public and private spaces.
Facial Recognition and Biometric Surveillance
Facial recognition technology has reached a level of accuracy and scalability that enables mass surveillance with unprecedented efficiency. Systems can identify individuals in crowds, track movements across time and space, and correlate this information with other data sources.
In China, an extensive network of AI-powered cameras monitors public spaces, with facial recognition systems able to identify individuals and track their movements. Similar technologies are being deployed globally, often with limited transparency or regulatory oversight. The privacy implications are far-reaching, potentially creating a world where anonymity in public becomes impossible.
Workplace Monitoring
AI tools are increasingly used to monitor employee productivity and behavior. Keystroke logging, email scanning, video analysis of work patterns, and other surveillance techniques create detailed profiles of employee activities that would have been impossible to compile manually.
During the COVID-19 pandemic, remote work monitoring software saw a dramatic increase in adoption. These tools can track active work hours, take periodic screenshots, monitor application usage, and even use webcams to verify presence. The level of scrutiny possible with AI-enhanced monitoring raises serious questions about workplace privacy and the psychological impacts of constant surveillance.
Algorithmic Bias and Discrimination
Privacy concerns extend beyond data collection to how AI systems process and act upon that data. Biased algorithms can create privacy harms by treating certain groups differently or exposing sensitive characteristics.
Disparate Impact on Marginalized Groups
AI systems often perform differently across demographic groups, potentially exposing certain populations to greater privacy risks. Facial recognition systems, for instance, have shown higher error rates for women and people with darker skin tones, which can lead to misidentification and unjust scrutiny.
When the New York Department of Education used an algorithm to evaluate teachers in 2015, the system produced wildly inconsistent results, with some teachers’ scores varying by as much as 80 percentile points from year to year. This unpredictability highlighted how algorithms could expose individuals to scrutiny based on flawed assessments rather than actual performance.
Amplification of Existing Inequalities
AI systems trained on historical data often reproduce and amplify existing social inequalities. This creates privacy concerns because individuals from marginalized groups may find their personal information subjected to greater scrutiny or misinterpreted through biased algorithmic lenses.
Credit scoring algorithms, for example, may incorporate factors that serve as proxies for protected characteristics like race, leading to discriminatory outcomes while exposing sensitive information about individuals. Similarly, healthcare algorithms have been found to prioritize care for white patients over Black patients with similar medical needs, creating both discrimination and privacy concerns.
Security Vulnerabilities
AI systems introduce new security vulnerabilities that can compromise privacy even when data protection measures are in place.
Adversarial Attacks
Researchers have demonstrated that AI systems can be vulnerable to adversarial attacks—carefully crafted inputs designed to confuse or manipulate the AI’s behavior. These attacks can potentially extract private information or cause the system to reveal details about its training data.
In 2019, researchers demonstrated that they could extract training data from GPT-2, a predecessor to current large language models. By providing carefully constructed prompts, they were able to make the model reproduce verbatim text from its training data, potentially including personal information.
Model Inversion Attacks
Advanced techniques like model inversion attacks can sometimes allow attackers to reconstruct the data used to train an AI model. This creates the risk that private information incorporated into training datasets could later be extracted, even if the original data was thought to be secure.
In one concerning demonstration, researchers were able to reconstruct recognizable facial images from a facial recognition model, essentially reversing the training process to extract private visual information about individuals in the training dataset.
Regulatory Responses and Frameworks
As AI privacy concerns have grown more pronounced, regulatory frameworks have begun to evolve in response, though many argue they remain insufficient.
General Data Protection Regulation (GDPR)
The European Union’s GDPR includes provisions specifically relevant to AI, including restrictions on automated decision-making, requirements for data minimization, and the right to explanation. These rules have influenced global approaches to AI privacy but face implementation challenges given the complexity of modern AI systems.
Article 22 of GDPR gives individuals the right not to be subject to purely automated decisions that have significant effects, including profiling. However, the practical application of this right remains unclear, particularly when human oversight is nominal rather than meaningful.
Emerging AI-Specific Regulation
Various jurisdictions are developing AI-specific regulatory frameworks that address privacy concerns. The EU’s proposed AI Act categorizes AI systems based on risk levels and imposes stricter requirements for high-risk applications, including those that process personal data extensively.
In the United States, regulatory approaches remain fragmented, with some states like California implementing broader privacy laws that affect AI systems. The California Consumer Privacy Act (CCPA) and its successor, the California Privacy Rights Act (CPRA), include provisions related to automated decision-making and profiling that have implications for AI privacy.
Organizational Approaches to AI Privacy
Organizations deploying AI systems are increasingly adopting practices designed to address privacy concerns, though implementation varies widely.
Privacy by Design
Privacy by Design principles encourage organizations to incorporate privacy considerations throughout the development lifecycle of AI systems rather than treating privacy as an afterthought. This approach includes data minimization, purpose limitation, and building privacy-enhancing technologies directly into AI architectures.
Microsoft’s Responsible AI principles include privacy commitments that influence how they design and deploy AI systems. This includes minimizing data collection, providing transparency about data use, and implementing technical safeguards to protect sensitive information.
Federated Learning and Differential Privacy
Technical approaches like federated learning allow AI models to be trained across multiple devices without centralizing sensitive data. The model travels to where the data is stored rather than vice versa, reducing privacy risks.
Google has implemented federated learning in products like Gboard, its mobile keyboard, to improve predictive text suggestions without sending users’ typing data to central servers. Similarly, Apple uses differential privacy techniques to collect aggregate statistics while adding mathematical noise to protect individual users’ data.
Individual Rights and Control
Empowering individuals with greater control over their data represents one approach to addressing AI privacy concerns, though significant challenges remain.
Right to Access and Erasure
Data protection laws increasingly provide individuals with rights to access information collected about them and request its deletion. However, the complexity of AI systems can make these rights difficult to implement effectively.
When individuals request data deletion under GDPR or similar laws, organizations may remove the direct data but struggle to eliminate all traces from trained AI models. The computational complexity of retraining models without specific data points makes true erasure technically challenging.
Privacy-Enhancing Technologies
Various technologies aim to give individuals more control over their data in AI-powered systems. Personal data stores, privacy-preserving computation, and user-controlled consent mechanisms all seek to rebalance power between individuals and AI systems.
Solid, a project led by web inventor Sir Tim Berners-Lee, proposes a decentralized approach where individuals store their data in personal “pods” and grant specific permissions to applications. This model aims to preserve privacy while still allowing AI systems to provide personalized services.
Future Directions and Emerging Challenges
As AI continues to evolve, new privacy challenges are emerging that will require innovative technical, regulatory, and ethical responses.
Multimodal AI and Comprehensive Profiling
Next-generation AI systems that process multiple types of data simultaneously—text, images, audio, video, biometrics—create more comprehensive profiles than ever before. These systems can draw connections across different aspects of individuals’ lives, potentially revealing patterns and insights that were previously impossible to detect.
Systems like GPT-4 with vision capabilities can analyze both text and images, potentially extracting personal information from visual content that users might not realize contains sensitive data. As these multimodal capabilities advance, the privacy implications grow more complex.
Synthetic Data and Privacy
Synthetic data—artificially generated information that mimics the statistical properties of real data without containing actual personal information—represents one potential solution to privacy concerns. However, questions remain about how effectively synthetic data can preserve utility while eliminating privacy risks.
OpenAI has explored using synthetic data to train certain aspects of its models, potentially reducing privacy risks associated with using real personal data. However, researchers continue to investigate whether synthetic data truly eliminates all privacy concerns or whether traces of the original training data may remain detectable.
Quantum Computing and Privacy
Looking further ahead, the development of quantum computing poses both threats and opportunities for AI privacy. Quantum capabilities could break current encryption methods, potentially exposing protected data, but could also enable new privacy-preserving computation techniques.
Researchers are already developing post-quantum cryptography methods designed to withstand attacks from quantum computers. These approaches will be essential for maintaining privacy as computational capabilities advance.
Conclusion
The privacy concerns surrounding artificial intelligence are multifaceted and evolving, touching on fundamental questions about control, consent, transparency, and power in the digital age. As AI systems become more sophisticated and ubiquitous, addressing these concerns will require coordinated efforts across technical, regulatory, organizational, and individual domains.
The path forward likely involves a combination of approaches: stronger regulatory frameworks that specifically address AI capabilities, technical innovations that enable privacy-preserving AI, organizational commitments to responsible practices, and enhanced individual rights and control mechanisms. By addressing privacy concerns proactively, we can work toward an AI ecosystem that delivers benefits while respecting fundamental rights to privacy and autonomy.
The ultimate challenge lies in finding the balance—harvesting the tremendous potential of AI to improve lives while ensuring these systems respect and protect the private sphere that remains essential to human dignity and freedom. This balance will not emerge automatically but must be deliberately crafted through thoughtful policy, innovative technology, and ongoing public engagement with these critical issues.